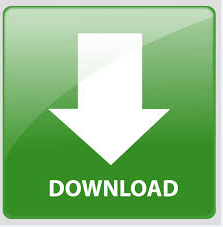
Recent advances in the field of computational social science have shown that the digital footprints people leave behind on a daily basis can be used to make accurate predictions about their psychological profiles (see e.g. They help us communicate with our friends and loved ones, capture the moments we care about the most, broadcast our opinions to millions of people around the world, search for information from the comfort of our homes, and pay for the things we want to buy with the ease of a tap or swipe. Over the past few decades, digital services and devices have become a central part of people’s everyday lives. Hence, for traits like Materialism, Self-control, Extraversion, and Neuroticism our findings could be used to improve psychologically-informed advertising strategies for specific products as well as personality-based spending management apps and credit scoring approaches. We found that Materialism and Self-Control could be inferred with relatively high levels of accuracy, while the accuracy obtained for the Big Five traits was lower, with only Extraversion and Neuroticism reaching reasonable classification performances. Our results show that inferring the psychological traits of an individual is a challenging task, even when using a comprehensive set of features that take temporal aspects of spending into account.
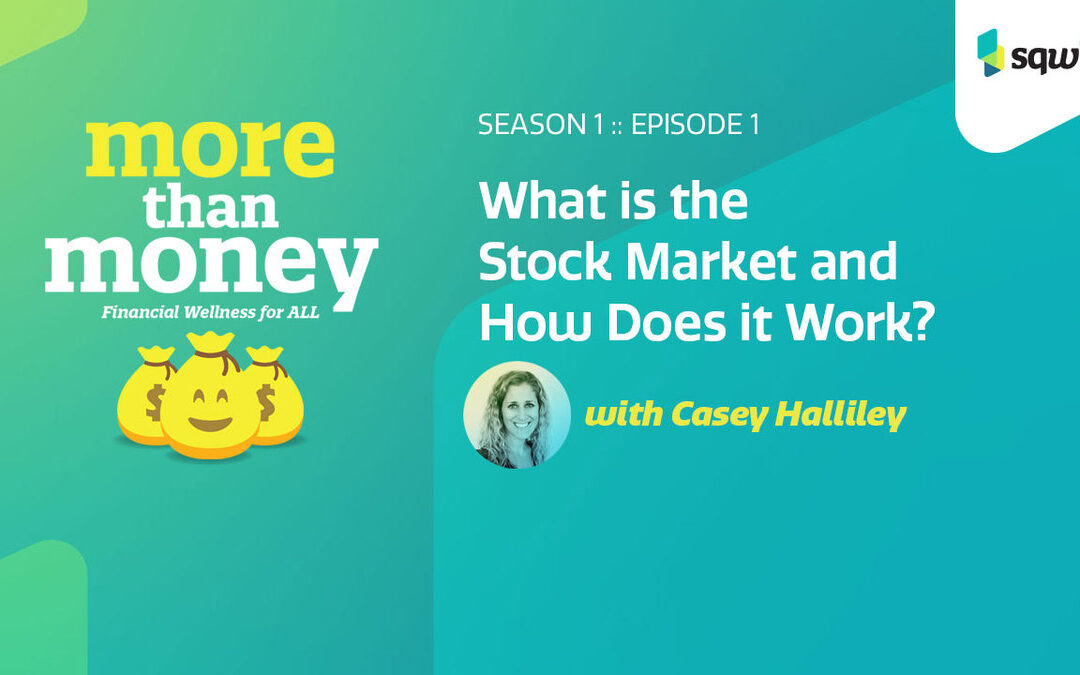
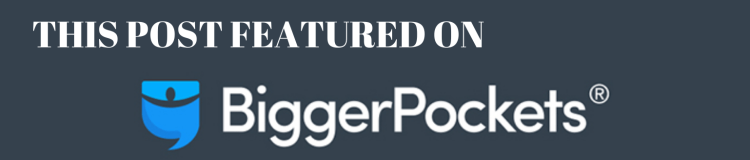
Using these features, we first explore their association with individual psychological characteristics, we then analyze the performances of the different feature families and finally, we try to understand to what extent psychological characteristics from spending records can be inferred. diversity, persistence, and turnover), (4) customer category profile, and (5) socio-demographic information. variability, persistence, and burstiness), (3) category-related spending behavior (i.e. total number and total amount of transaction), (2) temporal spending behavior (i.e. Specifically, we developed a more comprehensive feature space using: (1) overall spending behavior (i.e.
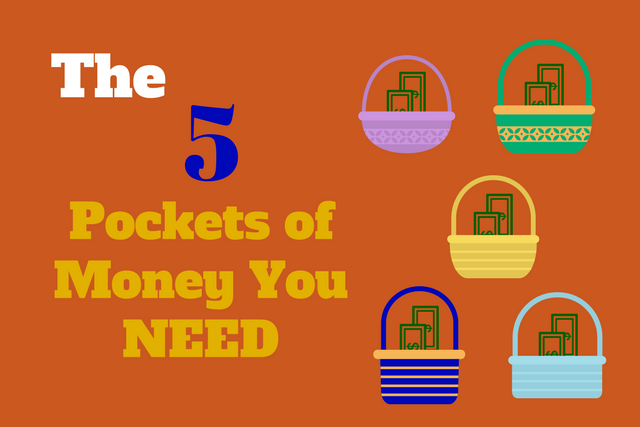
In this paper, using the transaction records of 1306 bank customers, we investigated the extent to which individual-level psychological characteristics can be inferred from bank transaction data. One type of digital footprint that is universal across the world, but that has received relatively little attention to date, is spending behavior. In recent years there has been a growing interest in analyzing human behavioral data generated by new technologies.
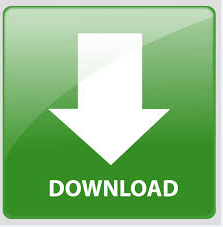